All published articles of this journal are available on ScienceDirect.
Predicting Readmission of Cardiovascular Patients Admitted to the CCU using Data Mining Techniques
Abstract
Background:
Cardiovascular (CV) diseases account for a large number of readmissions.
Objective:
Using data mining techniques, we aimed to predict the readmission of CV patients to Coronary Care Units of 4 public hospitals in Shiraz, Iran, within 30 days after discharge.
Methods:
To identify the variables affecting the readmission of CV patients in the present cross-sectional study, a comprehensive review of previous studies and the consensus of specialists and sub-specialists were used. The obtained variables were based on 264 readmitted and non-readmitted patients. Readmission was modeled with predictive algorithms with an accuracy of >70% using the IBM SPSS Modeler 18.0 software. Cross-Industry Standard Process for Data Mining (CRISP-DM) methodology provided a structured approach to planning the project.
Results:
Overall, 47 influential variables were included. The Support Vector Machine (SVM), Chi-square Automatic Interaction Detection (CHIAD), artificial neural network, C5.0, K-Nearest Neighbour, logistic regression, Classification and Regression (C&R) tree, and Quest algorithms with an accuracy of 98.60%, 89.60%, 89.90%, 88.00%, 85.90%, 79.90%, 78.60%, and 74.40%, respectively, were selected. The SVM algorithm was the best model for predicting readmission. According to this algorithm, the factors affecting readmission were age, arrhythmia, hypertension, chest pain, type of admission, cardiac or non-cardiac comorbidities, ejection fraction, undergoing coronary angiography, fluid and electrolyte disorders, and hospitalization 6-9 months before the current admission.
Conclusion:
According to the influential variables, it is suggested to educate patients, especially the older ones, about following physician advice and also to teach medical staff about up-to-date options to reduce readmissions.
1. INTRODUCTION
Increasing demand for health services, on the one hand, and limited resources, on the other hand, are major issues for health services. Therefore, it is suggested that health system policymakers and managers should aim for the optimal use oflimited resources available, including hospital beds, and increasing clinical effectiveness. One of the key indicators for assessing healthcare outcomes is the evaluation of readmission rates [1]. In general, readmission refers to rehospitalization within a specific time frame (usually within 30 days after discharge) in the same hospital or other ones on a planned or unplanned basis [2].
Currently, cardiovascular (CV) diseases account for a significant proportion of readmission cases, and a sharp increase in readmission rates for these diseases is worrying [3]. CV diseases are the most common cause of death in most countries, including Iran, and the leading cause of disability [4]. About 17.9 million people lost their lives in 2019 due to CV diseases, accounting for 32% of deaths worldwide [5]. Over three-fourths of the deaths from CV diseases occur in low- and middle-income countries [5], where people suffering from these diseases do not have adequate access to appropriate and effective hospital services, including Coronary Care Units (CCUs) [6, 7]. Therefore, today, there is an urgent need for CCUs more than ever, and this is why hospitals are seeking to increase the number of their CCU beds [8]. Discharge from this unit at the earliest appropriate time will reduce the cost of healthcare facilities and increase access to intensive care for critically ill patients. However, the early discharge of CCU patients may increase the likelihood of readmission and worsen the disease process [9].
Determining the factors affecting the readmission of CV patients will provide an opportunity to meet the needs of the patients and the problems related to the services provided. The results of numerous studies demonstrated that readmission of CV patients was affected by several factors, including demographic and social ones, such as age [10-13], gender [14, 15], type of health insurance coverage [16, 17], length of stay [18-21], type of admission [22, 23], discharge destination [19, 24-26], ethnicity [13, 27], and discharge status [25, 28], as well as some clinical factors like cardiac or non-cardiac comorbidities [29, 30], body mass index (BMI) [13, 31, 32], infection [33-36], fluid and electrolyte disorders [37, 38], surgery [13, 39], chest pain [40, 41], and depression [13, 42].
On the other hand, due to the large volume of available data and their complexity, the ever-increasing need for using an efficient, effective, and reliable tool for discovering useful knowledge in this data is required in order to determine the factors affecting readmissions. This goal can be achieved through the use of data mining algorithms. Data mining has good potential to create a knowledge-rich environment that can significantly increase the quality of clinical decisions [43]. Hence, some studies have used different data mining algorithms, such as logistic regression [44-48], support vector machine (SVM) [48-50], artificial neural network [47, 48], random forest [48, 49], and decision tree [48] to identify the factors affecting readmission of CV patients. However, in these studies, different findings have been reported.
Due to the diversity of techniques and their accuracy, authors used different data mining algorithms in the present study to identify the best algorithm for predicting the readmission of CV patients admitted to the CCUs of public hospitals in Shiraz, Iran, within 30 days after discharge in 2020.
2. MATERIALS AND METHODS
This was a cross-sectional study conducted on the patients admitted to the CCUs of public hospitals (4 hospitals) in Shiraz, Iran, in 2020 to predict the readmission of hospitalized CV patients through the use of data mining techniques. The Cross-Industry Standard Process for Data Mining (CRISP-DM) methodology was used to provide a structured approach to planning the data mining project [51]. As it was an iteration loop model, some steps might be performed several times to achieve the desired result in modeling. The CRISP-DM method included the following steps:
2.1. Business Understanding
At this stage, a comprehensive review of the articles in English on determining the factors affecting readmission of CV patients indexed in Web of Science, PubMed, Scopus, and Embase databases and published between 2000.01.01 and 2020.12.30 was carried out. The articles were searched using the following keywords:
“Factor OR Determinant OR Element” AND” Readmission OR Rehospitalization OR Re_admission OR Readmit OR Re_hospitalization” AND” Heart disease OR Cardiac disease OR Cardiovascular disease OR Cardiovascular patient OR Coronary artery disease OR Cardio surgeries OR Coronary artery bypass.
Finally, 45 variables were obtained. Then, using the consensus of 8 specialists and subspecialists working in the CCUs of the studied hospitals, 5 more variables were added, and a total of 50 variables were ultimately obtained (Appendices A and B).
2.2. Data Understanding
The patients who experienced readmission within 30 days after discharge were identified. The data relating to their demographic and clinical variables, the variables related to the service providers, and the data related to the variables were respectively obtained from the patients’ records, the Statistics unit of Shiraz University of Medical Sciences, and through interviews with the patients. The number of readmitted patients studied was equal to that of non-readmitted ones, and a total of 264 patients were examined. In other words, there were 132 readmitted patients, all of whom were examined through the census method. There were also 7362 non-readmitted patients in the four hospitals studied, of whom 132 were selected through the stratified random sampling method proportional to the size and simple random sampling method. The number of patients not readmitted to the CCUs of studied hospitals was determined based on the stratified random sampling method proportional to size. Within each hospital, these samples were selected through the simple random sampling method using their medical record numbers.
2.3. Data Preparation
At this stage, two variables of hospital ownership and being a teaching hospital were excluded from the study because all of the hospitals under study were teaching hospitals. In addition, the variable of training the patient on how to take medications was excluded due to the provision of education to all patients by nurses and the existence of the training sheet in all medical records. As a result, among the variables obtained from the comprehensive review of the articles and the consensus of the specialists and subspecialists, 47 variables were examined.
Moreover, since the body mass index (BMI) had not been recorded in some medical records, it was calculated through height and weight values extracted from these records.
Furthermore, a medical records technician classified principal diagnoses into seven main categories of CV diseases based on the International Classification of Diseases, Tenth Revision (ICD-10) (Appendix A).
2.4. Modeling
The readmission of the CV patients admitted to the CCUs of the studied hospitals within 30 days after discharge was odellin using the following algorithms through the use of the IBM SPSS Modeler 18.0 software:
C5.0 [52], logistic regression [53], Decision list [54], Discriminant [55], Bayesian Network [53], K-Nearest Neighbors (KNN) [56], Random Forest [53], Support Vector Machine (SVM) [56], Chi-square Automatic Interaction Detection (CHAID) [57], Quantile Estimation after Supervised Training (Quest) [58], Classification and Regression (C&R) Tree [58], and Artificial Neural Network (ANN) [59].
The algorithms with an accuracy of >70%, namely SVM, CHIAD, artificial neural network, C5.0, KNN, logistic regression, C&R tree, and Quest algorithms, were selected.
The input variables of these models included 47 identified variables.
Before odelling, the data were classified into three categories: training, testing, and validation. The validation data were used to check the overfitting of each model. The data from one of the hospitals, which included 49 patients (18.6%) (23 readmitted and 26 non-readmitted), were considered validation data and were not used in the training and testing stages. The data from the remaining three hospitals were randomly divided between the training (70%) and testing (30%) classes.
The calibration of the studied algorithms can be seen in Appendix C.
2.5. Evaluation
To evaluate the algorithms, the accuracy, sensitivity, specificity, positive predictive value, and negative predictive value measures were used, and to select the best algorithm for readmission prediction, the area under the Receiver Operating Characteristic (ROC) curve was also calculated.
3. RESULTS
The final 47 variables obtained from the comprehensive review of the published articles and the consensus of specialists and subspecialists are presented in appendices A and B. The values of accuracy, sensitivity, specificity, positive predictive value, and negative predictive value of each data mining algorithm used in the present study to predict readmission of CV patients are presented in Table 1 by training, testing, and validation data.
Model | Classification | Accuracy (%) | Sensitivity (%) | Specificity (%) | Positive Predictive Value (%) | Negative Predictive Value (%) |
---|---|---|---|---|---|---|
SVM algorithm | Training | 98.10 | 50.38 | 89.04 | 89.18 | 91.54 |
Testing | 97.80 | 52.38 | 90.90 | 91.66 | 88.33 | |
External validation | 100.00 | 100.00 | 100.00 | 100.00 | 100.00 | |
Mean | 98.60 | |||||
CHIAD algorithm | Training | 96.00 | 49.20 | 87.67 | 87.32 | 86.48 |
Testing | 78.90 | 53.06 | 69.69 | 72.22 | 67.64 | |
External validation | 93.90 | 50.00 | 85.84 | 85.84 | 83.48 | |
Mean | 89.60 | |||||
Artificial neural network algorithm | Training | 98.20 | 50.73 | 93.05 | 93.24 | 95.71 |
Testing | 82.10 | 61.53 | 60.60 | 71.11 | 83.33 | |
External validation | 89.40 | 42.94 | 92.38 | 90.12 | 73.48 | |
Mean | 89.90 | |||||
C5.0 algorithm | Training | 97.10 | 47.40 | 97.26 | 96.96 | 89.87 |
Testing | 76.00 | 53.70 | 75.75 | 78.37 | 75.75 | |
External validation | 91.00 | 52.10 | 85.84 | 86.84 | 90.09 | |
Mean | 88.00 | |||||
KNN algorithm | Training | 90.90 | 44.91 | 90.27 | 88.33 | 77.38 |
Testing | 74.90 | 46.93 | 78.78 | 76.66 | 65.00 | |
External validation | 91.90 | 54.94 | 78.09 | 81.30 | 90.10 | |
Mean | 85.90 | |||||
Logistic regression algorithm | Data set | 79.90 | 77.30 | 82.60 | 82.50 | 77.27 |
C&R tree algorithm | Training | 76.00 | 43.24 | 86.30 | 82.75 | 72.41 |
Testing | 73.70 | 50.00 | 81.81 | 81.81 | 72.97 | |
External validation | 86.10 | 55.74 | 72.64 | 76.98 | 86.51 | |
Mean | 78.60 | |||||
Quest algorithm | Training | 69.50 | 33.66 | 91.78 | 85.00 | 63.80 |
Testing | 75.50 | 34.46 | 96.96 | 95.23 | 65.30 | |
External validation | 78.30 | 45.45 | 84.90 | 82.41 | 72.58 | |
Mean | 74.40 |
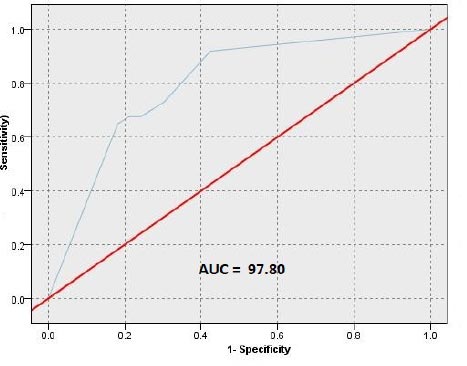
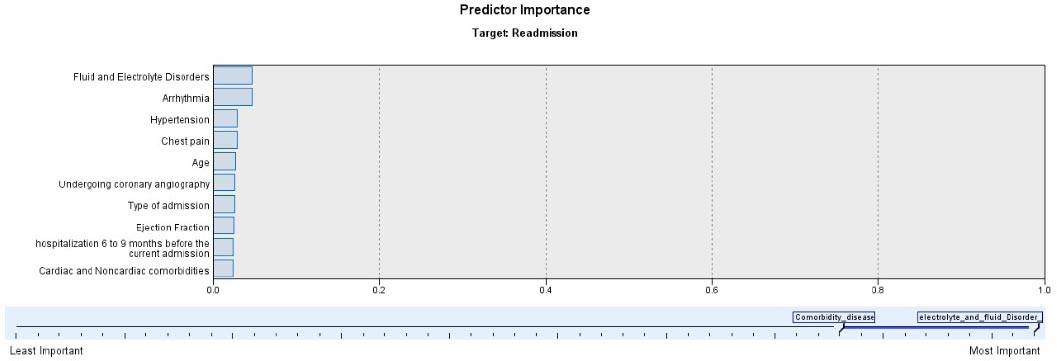
The results showed that the SVM algorithm was the best algorithm for predicting the readmission of CV patients (with 98.60% accuracy), while the Quest algorithm had the least accuracy (74.40%) (Table 1 and Fig. 1).
Based on the results of the SVM algorithm, the factors affecting the readmission of CV patients admitted to the studied CCUs were age, arrhythmia, hypertension, chest pain, type of admission (elective or non-elective), cardiac or non-cardiac comorbidities, ejection fraction, undergoing coronary angiography, fluid and electrolyte disorders, and hospitali- zation 6-9 months before the current admission (Fig. 2).
4. DISCUSSION
Readmission of CV patients is an important issue that can be investigated from various aspects, including the financial burden imposed on the patients and medical centers, the occupation of very valuable beds in the CCUs, and the lack of optimal use of facilities when providing services to CV patients [60]. This study aimed to predict the readmission for CV patients admitted to the CCUs of public hospitals in Shiraz, Iran, within 30 days after discharge in 2020 by using data mining techniques.
The results of the present study showed that the SVM algorithm, with an accuracy of 98.60%, was the best model for predicting readmission. The findings of the studies conducted by Yousefi et al. [61] and Zheng et al. [50] confirmed these results.
However, Golas et al. [62] reported that the accuracy of the deep unified networks (DUNs) model (76.4%) was higher than that of other models used to predict readmission of heart failure patients. In their cohort study, Wallmann et al. predicted the 30-day cardiovascular-disease-related readmissions from 2003 to 2009 at a Spanish academic tertiary care center using a predictive model developed by logistic regression and reported that the sensitivity, specificity, positive predictive value, and negative predictive value were 0.66, 0.7, 0.10 and 0.98, respectively [44]. Similarly, Desautels et al. conducted a study to identify patients who were likely to suffer unplanned ICU readmission at a single academic, tertiary care hospital in the UK from October 2014 to August 2016 on all patients who visited an ICU at some point during their stay using a logistic regression and showed that the accuracy of the logistic regression was 70.95% [45]. The results of these studies are inconsistent with those of the present study. Some reasons for the differences between the accuracy of the models in these studies and the present one can be differences in the population, hospital wards as well as the models used.
On the other hand, the study by Najafi-Vosough et al. indicated that the random forests algorithm was more accurate in predicting the readmission of heart failure patients than the SVM model [49]. The reason for the difference between their finding and that of the present study could be the difference in the type of variables and data studied. In their study, Futoma et al. compared data mining models to predict early hospital readmissions and suggested that the random forests and deep neural networks were better predictors of the readmission risk compared to the SVM algorithm [47], which is not in line with the results of the present study. The difference between the results could be due to the use of deep learning and some other techniques by Futoma et al. and the lack of using them in the present study. Another reason could be the difference in the studied populations (i.e., not only the patients with CV disease but also with obstructive pulmonary disease, pneumonia, and knee arthroplasty).
Awan et al. used machine learning methods to predict the readmission of heart failure patients and performed their modeling with the multilayer perceptron, logistic regression, random forest, decision tree, and support vector machine models. In their study, the multilayer perceptron model was selected as the best with the highest accuracy [48]. The reasons for the difference between the results of their study and the present research could be the imbalance of the data in their study as well as the difference in the studied populations (i.e., patients with a mean age of 81 years).
According to the results of the present study, the factors affecting readmission in the SVM algorithm included fluid and electrolyte disorders, arrhythmia, hypertension, chest pain, age, undergoing coronary angiography, type of admission (elective and non-elective), cardiac ejection fraction percentage, hospitalization 6-9 months before the current admission, and cardiac or non-cardiac comorbidities.
The present study indicated that fluid and electrolyte disorders were an effective factor in the readmission of CV patients. This is in line with the results of the studies conducted by Ranna et al. [38], Vogel et al. [27], and Lima et al. [11]. Due to the reduced cardiac ejection fraction percentage in patients with systolic heart failure, blood flow to the kidneys is also reduced, and kidney function is usually impaired. Kidney dysfunction in patients with heart failure is associated with the worsening of the symptoms of the disease and an increase in the length of hospital stay [63].
Also, usually, a large number of patients with CV diseases experience atrial fibrillation after heart surgery and are prone to persistent arrhythmias after discharge [64]. Therefore, it could be concluded that postoperative atrial fibrillation is common, and this is in line with the results of the studies conducted by Maniar et al., Iribarne et al., Arora et al., and Cedars et al. [34, 36, 65, 66].
In the present study, hypertension was identified as a factor affecting readmission, which is consistent with the results of the studies conducted by Kim et al. [67] and Bavishi et al. [68]. Hypertension is known as a gradual and quiet killer that can cause diseases, such as stroke. Due to the critical condition of patients studied in the present study, readmission is very common among people who also have hypertension. However, it is not in line with the results of the studies by Aranda Jr et al. [69] and Ferraris et al. [70], who stated that hypertension during hospitalization had an inverse relationship with readmission. The difference in the results could be due to differences in the studied populations and samples. Aranda et al. studied a population consisting of Medicare patients from the Centers for Medicare and Medicaid Services, and Ferraris et al. studied patients who underwent heart surgery.
Chest pain is one of the main symptoms of CV diseases [71] and is an effective factor in the readmission of CV patients in the present study. Given that chest pain can be a warning sign of cardiac ischemia, lack of adequate care for such patients might cause recurrent pain and readmission. The studies by Pellitier et al. [40] and Jarvinen et al. [41] confirmed the results of the present study.
The present study suggested that age was one of the factors affecting the readmission of CV patients. The results of the studies by Benuzilla et al. [72] and Bavishi et al. [68] confirmed this finding. Older people might not have all of their medical needs met before discharge. In addition, they may suffer from comorbidities and need more support and care in the community, and if this is not taken into account, they will be more likely to be readmitted.
The results of the SVM algorithm in the present study showed that undergoing coronary angiography was an influential factor in readmission because coronary angiography could cause complications. The femoral approach was the standard access site for coronary angiography, the use of which for angiography might cause complications, such as bleeding, hematoma, and embolism of the lower parts of the catheter entrance, due to the trauma to the artery wall [73, 74]. These complications could lead to readmission. The results of a study by Dunlay et al. confirmed these findings [75].
Based on the results of the present study, comorbidities (either cardiac or non-cardiac) are a factor determining the readmission of CV patients. It might be concluded that due to the lack of complete management and control of comorbidities and insufficient attention to them by patients and the severity of these diseases, CV patients with comorbidities usually need hospital care and readmission. According to the findings of previous studies, non-cardiac comorbidities [26, 76-83] and cardiac comorbidities [17, 20, 26, 68, 85-88] have been factors influencing the readmission of CV patients.
The present study had some limitations, including its cross-sectional design, small sample size as well as the non-use of complete pharmacological and laboratory data of the studied patients.
CONCLUSION
According to the results of the present study, SVM was the best algorithm in terms of accuracy. Based on the results of this algorithm, the factors affecting the readmission were age, arrhythmia, hypertension, chest pain, type of admission (elective and non-elective), cardiac or non-cardiac comorbidities, ejection fraction, undergoing coronary angiography, fluid and electrolyte disorders, and hospitalization 6-9 months before the current admission. Therefore, it is recommended to teach the patients, especially the older ones, about the regular use of the drugs and following physician advice after discharge. It is also suggested to train medical staff about the relevant and up-to-date options in order to reduce the likelihood of patient readmissions.
AUTHOR CONTRIBUTIONS
RR, PB, and MN designed the study. MS collected the required data. MK and MS analyzed the data. All authors interpreted the data. RR and MS wrote the first draft of the manuscript. All authors reviewed and edited the manuscript and approved the final version of the manuscript.
ETHICS APPROVAL AND CONSENT TO PARTICIPATE
This study was approved by the Shiraz University of Medical Sciences Ethics Committee (Code: IR.SUMS.REC.1398.272).
HUMAN AND ANIMAL RIGHTS
No animals were used in this research. All procedures performed in studies involving human participants were in accordance with the ethical standards of institutional and/or research committee and with the 1975 Declaration of Helsinki, as revised in 2013.
CONSENT FOR PUBLICATION
To participate in this study, all the patients gave their written informed consent, and all were assured of the confidentiality of their responses.
STANDARDS OF REPORTING
STROBE guidelines were followed.
AVAILABILITY OF DATA AND MATERIALS
The datasets used and/or analyzed during the current study are available from the corresponding author [R.R] upon reasonable request.
CONFLICT OF INTEREST
The authors declare no conflict of interest.
FUNDING
The present article was extracted from the thesis written by Marzie Salimi and was financially supported by Shiraz University of Medical Sciences (grant No. 97-01-07-18833).
ACKNOWLEDGMENTS
The present article was extracted from the thesis written by Marzie Salimi and was financially supported by Shiraz University of Medical Sciences grant No. 97-01-07-18833. The researchers would like to thank the studied patients for their kind cooperation with the researchers in collecting and analysing the data.
APPENDIX
Variables | Data variable categories | Variable definition | Type | References | Variable | Data variable categories | Variable definition | Type | References | ||
---|---|---|---|---|---|---|---|---|---|---|---|
1 | Gender | Female = 0 | Male & female | Input | [14, 15] | 22 | Having cardiac or non-cardiac comorbidities | No = 0 | Having cardiac or non-cardiac comorbidities at the time of admission | Input | [29, 30, 89] |
Male = 1 | Yes = 1 | ||||||||||
2 | Marital status | Married = 0 | Patient’s marital status at the time of admission to the CCU | Input | [29, 90] | 23 | Having peripheral artery diseases | No = 0 | Diseases that affect blood vessels outside the heart and brain, such as the arms, legs, etc. | Input | [83] |
Single = 1 | Yes = 1 | ||||||||||
3 | Education level | Illiterate = 0 | Patient education level at the time of admission to the CCU | Input | [91] | 24 | Having chest pain | No = 0 | Having chest pain, according to the patient's report and the contents of the patient’s medical records | Input | [40, 41, 68, 92] |
Elementary = 1 | Yes = 1 | ||||||||||
Middle school = 2 | 25 | Having depression | No = 0 | Having patient depression, according to the physician's diagnosis and the patient’s medical records | Input | [42] | |||||
Diploma = 3 | Yes = 1 | ||||||||||
Academic education = 4 | 26 | Pregnancy | No = 0 | Being pregnant, according to the patient’s report and the contents of the patient’s medical records | Input | [89] | |||||
Yes = 1 | |||||||||||
4 | Occupation | Self-employed = 0 | Being self-employed, housewife, employee, or retired | Input | [92] | 27 | Having infection | No = 0 | Having patient infection at the time of admission to and during hospitalization in the CCU | Input | [33-36] |
Housewife = 1 | Yes = 1 | ||||||||||
Employee = 2 | 28 | Undergoing dialysis | No = 0 | Having undergone dialysis at the time of admission to the CCU | Input | [32] | |||||
Retired = 3 | Yes = 1 | ||||||||||
5 | Housing status | Owner = 0 | Being an owner or tenant at the time of admission to the CCU | Input | [93] | 29 | Poor right ventricular function | No = 0 | Poor right ventricular function according to the echocardiographic results | Input | [94] |
Tenant = 1 | Yes = 1 | ||||||||||
6 | Governmental health insurance coverage | No = 0 | Having governmental health insurance coverage | [17, 16] | 30 | Undergoing coronary angiography | No = 0 | Having or not having undergone coronary angiography at the time of hospitalization | Input | Specialists and sub-specialists consensus | |
Yes = 1 | Input | Yes = 1 | |||||||||
31 | Having arrhythmia | No = 0 | Having abnormal heart rhythm | Input | [30] | ||||||
7 | Place of residence | Urban area = 0 | Being a resident of a city or a village | Input | [95] | Yes = 1 | |||||
Rural area = 1 | 32 | Training the patient on how to take medications | No = 0 | Existence of the drug use instruction sheet in the patient’s medical records | Input | [92] | |||||
8 | Nationality | Iranian = 0 | Being Iranian or a foreigner | Input | [13, 27] | Yes = 1 | |||||
Foreigner = 1 | 33 | Prescribing special medicines | No = 0 | Type of special medicines prescribed by physicians for after discharge, such as beta-blockers and anticoagulants | Input | [29, 79, 96] | |||||
9 | Taking herbal medicines | No = 0 | Taking herbal medicines after discharge | Input | Specialists' and sub-specialists' consensus | Yes = 1 | |||||
Yes = 1 | 34 | Fluids and electrolyte disorders | No = 0 | Disorders of factors, such as creatinine, potassium, etc. | Input | [37, 38] | |||||
10 | Following a special diet suitable for cardiovascular patients | No = 0 | Following a special diet suitable for cardiovascular patients after discharge | Input | Specialists' and sub-specialists' consensus | Yes = 1 | |||||
Yes = 1 | 35 | Hematocrit level | Normal = 0 | Normal: 40% to 52% Abnormal: <40 | Input | [39, 97] | |||||
11 | Discharge on weekend | No = 0 | Discharge on the weekend (Thursday and Friday) | Input | [98, 99] | Abnormal = 1 | |||||
Yes = 1 | |||||||||||
12 | Destination after discharge | Home = 0 | Destination of patients after discharge from hospital (home or other medical centers or non-medical centers, such as nursing homes) | Input | [19, 24-26] | 36 | Hemoglobin levels | Normal = 0 | Normal: 12 to 16.5 g/dl | Input | [36, 100, 101] |
Other medical centers = 1 | Abnormal = 1 | ||||||||||
Non-medical centers = 2 | |||||||||||
13 | Type of admission | Elective = 0 | Patient status at the time of admission | Input | [22, 23] | 37 | Red blood cell levels | Normal = 0 | Normal: 4.50 to 5.90 ml / m3 | Input | [102] |
Non-elective (emergency) = 1 | Abnormal = 1 | ||||||||||
14 | Discharge season | Spring = 0 | The season when the patient was discharged | Input | |||||||
Summer = 1 | [103] | 38 | Blood cholesterol levels | Normal = 0 | 200 mg / dL is normal, and above this value is abnormal. | Input | [103] | ||||
Fall = 2 | Abnormal = 1 | ||||||||||
Winter = 3 | |||||||||||
15 | Hospitalization 6 to 9 months before the current admission | No = 0 | Hospitalization 6-9 months before the current admission | Input | [79, 92, 98] | 39 | Principal diagnoses | Cardiac ischemia = 0 | Principal diagnoses by physicians at the time of admission to the hospital | Input | Specialists' and sub-specialists' consensus |
Yes = 1 | Other heart diseases = 1 | ||||||||||
16 | Physician’s Certificate | Specialist = 0 | Under-treatment by a specialist or a sub-specialist | Input | [104] | Cerebrovascular diseases = 2 | |||||
Sub-specialist = 1 | Blood pressure diseases = 3 | ||||||||||
17 | Hospital ownership | Public = 0 | Being public or private | Input | [105] | Chronic rheumatic disease = 4 | |||||
18 | Type of hospital | Teaching = 0 | Being teaching or non-teaching | Input | [106] | Complications of prostheses, implants, and grafts = 5 | |||||
19 | Having hypertension | No = 0 | Blood pressure> 14 mm Hg | Input | [27, 68] | Abnormal clinical signs not classified elsewhere = 6 | |||||
Yes = 1 | 40 | Heartbeat | Normal = 0 | Normal: 60 to 100 beats/ min | Input | [107] | |||||
Abnormal = 1 | |||||||||||
41 | Smoking | No = 0 | Consumption of hookah, cigarette, etc. | Input | [87, 108] | ||||||
20 | Having hyperlipidemia | No = 0 | Increased levels of lipids in the blood | Input | [109] | Yes = 1 | |||||
Yes = 1 | 42 | Discharge status | With the physician’s permission = 0 | Discharge with physician’s permission or voluntarily with personal consent | Input | (23, 24 | |||||
21 | Readmission | No = 0 | The patient returns to the hospital within 30 days after discharge | Target | [1, 2] | Voluntarily with personal consent =1 | |||||
Yes = 1 | 43 | Surgery | No = 0 | Having undergone heart surgery during hospitalization | Input | [13, 39] | |||||
Yes = 1 |
Reference | Variable definition | Variable | |
---|---|---|---|
[10-13] | Patient’s age at the admission time | Age | 1 |
[105] | Patient’s weight at the admission time | Weight | 2 |
[18-21] | Length of stay in hospital | Length of stay | 3 |
[53] | Number of active and available hospital beds | Hospital bed | 4 |
[110] | The ratio of nurses to the CCU beds | The ratio of nurses to the CCU beds | 5 |
Specialists' and sub-specialists' consensus | The ratio of physicians to the CCU beds | The ratio of physicians to the CCU beds | 6 |
[13, 31, 32] | kg/m2, where kg is a patient’s weight in kilograms, and m2 is his/her height in meter square | Body Mass Index | 7 |
[15, 86] | It is a measure used by physicians to calculate the percentage of blood coming out of the ventricles in each contraction. | Ejection fraction (EF) percentage | 8 |
Model | Specification | Value | Model | Specification | Value |
---|---|---|---|---|---|
SVM | Use partitioned data | True | KNN | Use partitioned data | True |
Partition | Partition | Partition | Partition | ||
Stopping criteria | 1.0E-03 | What type of analysis do you want to perform? | Predict a target field | ||
Kernel type | RBF | What is your objective? | Balance speed and accuracy | ||
Regularization parameter | 10 | Focal face value | 0 | ||
Regression precision (ε) | 0.1 | Normalize range input | True | ||
RBF gamma | 0.1 | Distance computation | Euclidean metric | ||
Gamma | 1 | Prediction for range target | The mean of the nearest neighbor value | ||
Bias | 0 | Automatically select K | True | ||
Degree | 3 | K | 3 | ||
Algorithm | SVM | Minimum | 3 | ||
Model type | Classification | Maximum | 5 | ||
NN | N Neural network model | Multilayer perceptron (MLP) | Number to select | 10 | |
Hidden layer | Automatically compute the number of units | Minimum change | 0.01 | ||
Hidden layer 1 | 1 | Number of folds | 10 | ||
Hidden layer 2 | 0 | C&R Tree | Use partitioned data | True | |
Random seed | 229176228 | Partition | partition | ||
Replicate results | True | Calculate predictor importance | True | ||
Minute | 15 | Level below root | 5 | ||
Use maximum training time (per component model) | True | Model | Expert | ||
Overfit prevention set (%) | 30 | Maximum surrogate | 5 | ||
Missing value in predictors | Delete list wise | The minimum change in the impurity | 0.0 | ||
Method | Neural network | Impurity measure for categorical target | Gini | ||
CHAID | Use partitioned data | True | Stopping criteria | Use percentage | |
Partition | Partition | Minimum records parents branch (%) | 2 | ||
Calculate predictor importance | True | Minimum records child branch (%) | 1 | ||
Method | CHAID | Prune tree | True | ||
Level below root | 5 | Prior probabilities | Based on training data | ||
Alpha for splitting | 0.05 | QUEST | Use partitioned data | True | |
Alpha for merging | 0.05 | Partition | Partition | ||
Epsilon for convergence | 0.001 | Calculate predictor importance | True | ||
Use Bonferroni adjustment | true | Level below root | 5 | ||
Maximum iterations for convergence | 100 | Mode | Expert | ||
Chi-square method | Pearson | Maximum surrogates | 5 | ||
Stopping criteria | Use percentage | Alpha for splitting | 0.05 | ||
Minimum records parents branch (%) | 2 | Stopping criteria | Use percentage | ||
Minimum record child branch (%) | 1 | Minimum records parents branch (%) | 2 | ||
C5.0 | Use partitioned data | True | Minimum record child branch (%) | 1 | |
Partition | Partition | Prune tree | True | ||
Calculate predictor importance | True | Prior probabilities | Based on training data | ||
Output type | Decision tree | Algorithm | QUEST | ||
Mode | Simple | Model type | Classification | ||
Favor | Accuracy | ||||
Tree depth | 10 | ||||
Expected noise (%) | 0 | ||||
Model type | Classification |